AIASC 210: Balance Sheet for AI Assets and Liabilities
Purpose and Scope:
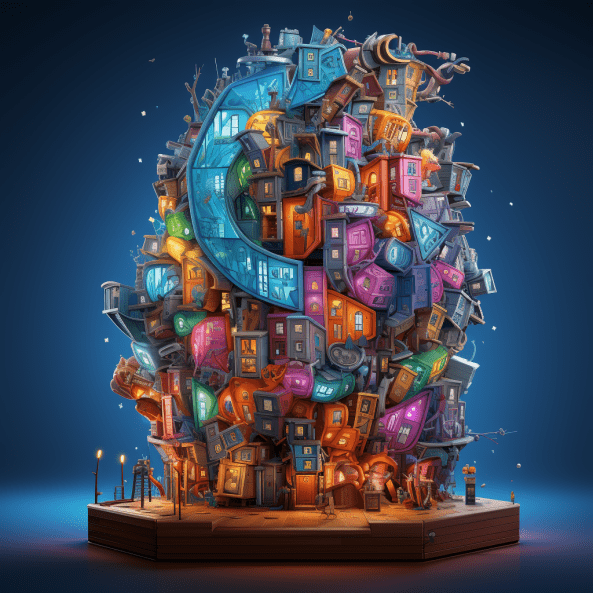
This document provides guidance on presenting assets and liabilities specifically related to artificial intelligence on an entity's balance sheet. The principles ensure that stakeholders have a clear and consistent view of an entity's AI investments and obligations.
1. Principle of AI Asset Classification:
- AI assets, such as trained models, proprietary algorithms, and related intellectual property, should be clearly identified and classified separately from traditional intangible assets.
2. Principle of Valuation:
- AI assets should be valued based on a combination of their development costs, future earning potential, and any external benchmark or market-based valuations, if available.
3. Principle of Data Asset Recognition:
- Data sets used for training AI models, if proprietary and having future economic benefits, should be recognized as separate assets, with clear valuation methodologies.
4. Principle of AI Liabilities:
- Liabilities arising from AI, such as potential litigations from biased decisions or breaches in data used by AI, should be recognized and measured appropriately.
5. Principle of Depreciation and Amortization:
- Given the rapid evolution of AI technologies, AI assets might have different useful lives. The method and period of depreciation or amortization should reflect the asset's expected economic benefit period.
6. Principle of Continuous Reassessment:
- The value of AI assets and liabilities should be continuously reassessed, given the dynamic nature of technology and its applications.
7. Principle of Disclosures:
- Significant assumptions used in the valuation of AI assets and liabilities, as well as any risks associated with them, should be disclosed transparently.
Updates and Amendments:The AIASC 210 guidelines will be periodically reviewed and updated to consider advancements in AI technology, valuation methods, and feedback from stakeholders and the public.
Note: This is a fictional representation and does not represent any real-world standard for AI. The development of such standards would involve extensive consultations with experts, stakeholders, and the public. Fictional representations simplify complex AI concepts, stimulate discussion, envision future scenarios, highlight ethical considerations, encourage creativity, bridge knowledge gaps, and set benchmarks for debate in fields like accounting.